1. Introduction
Clear and intelligible speaking is an important skill for active and successful communication in daily conversations. Speech intelligibility is also an important issue for both fire alarm system designers and speech-language therapists, let alone language teachers. A lack of an intelligible emergency voice alarm could lead to personal losses from apartment fires. General intelligibility testing is conducted by both subject-based word and rhyme tests by panels of listeners and by quantitative methods using common intelligibility scales (Nolan, 2012). Conversely, speech therapists help speakers with speech sound disorders to attain and maintain intelligible speech (Miller, 2013). Therapists rely on valid and reliable assessments for providing a basis for the best clinical decision making and monitoring. In language teaching, learners’ pronunciation plays an important role in delivering intelligible speech. Levis & LeVelle (2011) noted in an overview of a second language learning and teaching conference that a current goal of pronunciation teaching generally aims at helping learners to achieve comfortably intelligible pronunciation. According to Smith & Nelson (2006), intelligibility is concerned with the word and utterance level of recognition, while comprehensibility goes further to the meaning of the word, and interpretability goes to the implicit messages of the speaker. From these studies we may define intelligibility as the degree to which a message is heard as intended. In the past, Korean English learners aimed to achieve a native like proficiency level, but the majority of them failed to do so. Korean learners would rather acquire intelligible pronunciation by appropriate understanding of both the English and Korean sound systems, followed by an absolute number of practice hours.
After a certain period of teaching, a valid and reliable evaluation of pronunciation would be quite helpful to diagnose and remedy certain chronic problems of individual learners, just like the speech therapists’ practice mentioned above. The evaluation of pronunciation can be performed in global aspects, as well as in local features. Levis (2011) at the second language learning and teaching conference noted that judgments of speech intelligibility can be influenced by a variety of features, including listener-specific factors, foreign accents, or the use of read or free speech. For example, listeners might listen more effectively once they are familiar with the particular accents and error patterns of a given talker. The panelists in a discussion of Korean and Spanish talkers’ read speech at the conference were quite critical of the evaluation of intelligibility simply because read speech sounded like a strange and unnatural activity, except for reading to the children. The speakers often did not focus on the meaning of the text, which led to inappropriate parsing errors. The panelists noted a problem of using a written text to assess intelligibility unless the speakers were given sufficient preparation in the task. Currently, most Korean college English teachers are in charge of large classes that exceed their capacity. Thus, they tend to avoid any pronunciation evaluation that might require tremendous time and effort in listening to and evaluating students’ recordings of home assignments. Korean teachers might ask native English colleagues through a co-teaching plan to take over the heavy duty of the evaluation or rely on an evaluation application for this purpose.
Not much research has reported on the use of speech recognition applications for the evaluation of intelligible speech. Yang (2017) attempted to use Google speech recognition to examine college students’ pronunciation of a short English paragraph and concluded that the speech recognition application was useful for diagnosing learners’ specific pronunciation problems. The recognition rates have varied depending on speech modes. Specifically, the clear speech mode yielded a 10% greater recognition rate than the casual speech mode in the study. In addition, Yang reported several errors of the application itself, such as the fricative sounds produced by both native English speakers and Koreans. In contrast, Kang & Ahn (2013) asked thirty Korean students to produce a list of words and sentences including picture story telling. Five native English raters listened to the words and judged the accuracy of the English [r] sound and the intelligibility of the speakers’ speech on a nine-point scale. They also measured F3 values at the onset point of [r] rising to compare their ratings. They found no significant relationship between the students’ proficiency levels and the raters’ accuracy evaluations and suggested focusing more on an intelligibility-based pronunciation education. In addition, they proposed providing the raters with clear definitions of accuracy and intelligibility norms because some raters were confused.
Loukina et al. (2015) investigated the L2 speech corpus of 143 nonnative speakers to examine the connection between perceived intelligibility and pronunciation accuracy. The speakers listened to recorded conversations or lectures and were prompted to talk for one minute. Then, fifty-seven native annotators transcribed the content words of the non-native productions because short function words of even native speakers’ production were not recognized correctly. They also identified the nonnatives’ pronunciation errors, and they reported that 46% of all keywords of nonnative productions were recognized by native listeners. The researchers reported that mispronunciations by the nonnative speakers only predicted a small amount of the variance in intelligibility, and the full model of intelligibility should consider context-related effects, as well as pronunciation accuracy. They proposed using an automatic system that objectively evaluated intelligibility levels because the proficiency score by the raters might not reflect the intelligibility sufficiently accurately.
The main purpose of this study was to contribute to the possible application of a speech recognition application to an evaluation of pronunciation of an English passage by Korean students. Specifically, the current study was designed to investigate the relationship between speech recognition rate and human evaluation of English pronunciation and to provide fundamental data for potential speech scientists and English teachers.
2. Methods
Thirty-one college and graduate students participated in the recording of a short English passage. They were divided into nineteen undergraduate and twelve graduate students. They majored in English education and took a course in applied phonetics before the data recording. Their English proficiency varied, but a majority of them were assumed to be at an intermediate or higher level. Two native English raters were recruited to evaluate the students’ recordings. They were Canadians currently teaching college English, and they had much experience evaluating Korean students’ pronunciations. One had a teaching career with students for more than eleven years in Korea and the other fifteen years.
The recording passage was obtained from a website (Rochester Institute of Technology, 2020). The rainbow passage consisted of 98 words and six sentences as follows.
-
When the sunlight strike s
-
theraindrops in theair,
-
they act like a prism
-
andformarainbow.
-
The rainbow is a division of white light
-
into many beautiful color s.
-
Thesetaketheshapeof along round arch,
-
with its path high above,
-
and itstwo endsapparentlybeyond thehorizon.
-
Thereis, accordingtolegend,
-
aboiling potofgoldatone end.
-
People look , but no one ever find s it.
-
When aman looks for something beyond hisreach,
-
his friend s say he is look ing for the pot of gold
-
at theendof therainbow.
To use the string comparison “equal” function in Microsoft Excel, each sentence was parsed into fifteen numbered rows within which the same word did not occur. According to two major groups of parts of speech, forty-seven content words were written in bold face, while fifty-one function words, as well as plural morphemes (-s, raindrops, colors, ends, friends) and inflectional morphemes (-s, strikes, finds), were denoted in plain type (see Benner, 2020; Nordquist, 2020; O’Shea, 2013 for group classification).
Data for analysis were collected in three steps: recording of the rainbow passage by the participants at home using their mobile phones; recognition of the recording by a speech recognition application, Speechnotes (https://speechnotes.co/); and evaluation of the recording by two native Canadian English-speaking raters. The application uses Google’s speech-recognition engines with expected accuracy levels higher than 90%, which was proved by the two Canadians’ recordings. Statistical analyses of the recognition rate and human raters were conducted using Microsoft Excel and R software (R Core Team, 2020).
To avoid the reader’s parsing errors without knowing the context of the passage, the author asked the participants to prepare for the task by listening to the native pronunciation of the passage using Papago (Naver, 2020) and by practicing it sufficiently in advance (Levis, 2011). As mentioned in the introduction, there might have been some evaluation problems regarding authenticity in read speech. However, we decided to use the passage to ensure comparable recordings in clear speech mode. If we used free speech, recognition rates and intelligibility levels on speech recordings of different sizes, word choices, or syntactic structures might not be comparable among various participants. Further studies of both free and read speeches are desirable to pursue any findings related to the comparability issue.
The original recordings of the participants were converted to the same file format, i.e., 16 bit mono at a 44.1-kHz sampling rate, and normalized to the peak of the sound files. Some speech segments with low intensity were boosted by SoundStudio on a Macintosh computer to render the sounds sufficiently loud for the speech recognition application and the human raters. Then, the sound files were played on the computer, and the recognized output texts were transcribed by the speech recognition application on a Samsung Galaxy Note 10 in a quiet room. The recognition procedure was applied twice per each speaker in a row. There were slight differences in the transcribed output texts. The recognition rate was calculated by determining the percentage of correctly recognized words, which occurred in either the first or second output texts. The recognized words were also divided into forty-seven content words and fifty-one function words to examine any group differences in the word types. Both the content words with and without the morphemes were counted as correctly recognized words in this paper. The “table” function in R was used to collect the categorical frequency distribution of unrecognized words or general patterns of incorrect recognition.
The two native raters listened to the recordings and evaluated the read speech of the thirty-one participants using International English Language Testing System (IELTS) band criteria for pronunciation (IDP Education Canada, 2020). The speaking section in IELTS assesses the use of free spoken English through an examiner’s individual face-to-face interview on test takers’ homes, families, and interests in Part 1, and the test takers may write down their own thoughts on an assigned topic for a minute and answer them in two minutes in Part 2 and discuss them further in Part 3. The level is determined by the band descriptors from 0 to 9 for fluency and coherence, lexical resource, grammatical range, accuracy and pronunciation. The scoring description for a very good user of band 8 reads a wide range of pronunciation features and has an easy understanding throughout, as well as minimal effect of the L1 accent on intelligibility. The raters were instructed to evaluate the participants’ recordings using the full range focusing only on pronunciation intelligibility of the read speech. The raters watched a 15-minute sample video interview of five Korean IELTS test takers in five bands: 5.5, 6.5, 7.5, 8.5, and 9. In this way, they could avoid confusion regarding the intelligibility band reported in Kang & Ahn (2013).
3. Results and Discussion
Table 1 lists basic statistics of speech recognition rates by the speech recognition application. To facilitate the readers’ understanding, both the number of correctly recognized words and their percentages of the total number of words in the rainbow passage are reported in the table. We use mostly percentages in the discussion. As described in the previous section, the total number of words in the passage was 98: 47 content words and 51 function words. The grand average percentage of correctly recognized words was 77.7%, i.e., 76.2 of 98 words. Had we used a different recording passage, the result would have varied. The correctly recognized percentage of all of the words ranged from 63.3% to 87.8%. The moderate rate generally indicates the proficiency level of the participants as intermediate or higher. It also suggested the usefulness of the recognition application as an evaluation tool. Interestingly, the correctly recognized percentage of the function words was greater than that of the content words by approximately 8% points.
The percentage of the standard deviation of all correctly recognized words was approximately 6%. There was a 3.5% difference in the deviation between the content and function word groups. The content word group showed lower recognition and wider deviation, which might be related to some pronunciation errors of specific consonants or vowels by the participants. Conversely, the range of correctly recognized function words extended much wider than the content words. We examine frequently unrecognized words and pronunciation errors in the section below. The higher recognition rate of the function words might be related to hyperarticulation of the participants without applying such general phonological processes as resyllabification or vowel weakening to their read speech.
The participants were divided into high- and low-level groups greater and less than 79% of the recognition rate: fifteen high-level and sixteen low-level participants. Table 2 shows the group statistics of speech recognition rates of the content and function words.
The table indicates that the two groups are different in basic statistics. The average percentage of the high-level group is 82.8%, while that of the low-level group is 73.0%. The difference between the two groups amounts to 9.8%. From the maximum and minimum rates we determine the range of each group from 8.2% to 15.3%, respectively. Both the high- and low-level groups demonstrate higher recognition rates of the function words by 5.1% and 11.2%, respectively. The result might be related to the reliability of the recognition algorithm and recording devices. The recordings were made by the participants with their own mobile phones in various settings. Further studies might find it interesting to compare the results of sound-proof booths or individual recording settings. The standard deviation of the high-level group was almost half of the deviation of the low-level group. The difference might be related to the pool of the participants and the selection of the passage in this study. Different pools of each group and level of the passage would lead to various patterns of difficulties with the content and function words.
Table 3 illustrates the percentage of correctly recognized words by sentence type. One can note that the recognition rates vary by the size and order of the sentence. The best recognition occurs in the last sentence, while the third sentence lists the worst recognition rate. The last phrase in Row 15 records 100% recognition. Among the rows in the third sentence, we found 53.5% recognition in Row 8. The word “path” lists many unrecognized cases, and in Row 4, for the first sentence, the phrase “form a” listed an almost comparable low rate of 58.1%, in which the two words were recognized as one word like the English word “former”. We discuss a few cases of one-word recognition for a pair of words in the following section. In contrast, the first sentence records 72.8% recognition. The low recognition rate at the beginning might be related to abrupt adaptation of the speech recognition application itself. There used to be a short silence period at the beginning of the recording so that the application had sufficient sound data to immediately begin the recognition process. From these results, we could say that the recognition could vary depending on the composition of the content and function words in each given sentence. To perform a meaningful evaluation of the participants’ speech, we might have to examine controlled or balanced components of the vocabulary from both free and read speech (Levis, 2011).
An examination of the unrecognized words might be necessary to diagnose and remedy the talker’s pronunciation errors. Table 4 lists the frequency counts of unrecognized content and function words.
The content word “looks” ranks as the most frequently unrecognized instance, followed by the word “is”. The two words consist of one syllable, and they are frequently connected to adjacent words. In addition, typical pronunciation problems of the Korean participants seemed to be related to the lateral [l] sound and the lax vowel in the word “look”. The lateral must be produced like a Korean flap in casual production of the English word “water”. The lax vowel [ʊ] is also quite difficult for Koreans to realize sufficient vowel quality (Yang & Whalen, 2015). The words “form” and “path” ranked third and fourth. A few instances of the word “form” were wrongly recognized as “from”. Several instances of the word “path” were recognized as “pet, pat, pass, past, pets”. Both the vowel quality and the fricative features could account for the variants. The second to fourth words on the list have either a fricative onset or coda, which must have contributed to the low recognition rate. Yang (2017) reported the same recognition problem in a paragraph with words with fricative codas, such as “slabs” and “these”. He attributed the low recognition rate of casual speech to problems of individual pronunciation errors and Google soundwriter errors with the fricative sounds. Similar fricative problems could be found with the function words on the list. Yang also noted that the main error sources might be traced to the phonotactic probability (Vitevitch & Luce, 2004) and lexical neighborhood density (Luce & Pisoni, 1998) of the words in the paragraph.
The vowel in the word “ends” was recognized as “and, ann’s, as, hands,” and others. The quality of the vowel might have to be considered for better recognition. A study of the recognition rate of a separate list of minimal pairs of vowels for the same participants might be useful to explain partial causes of the total recognition rate of a passage, including either one or the other minimal pair of words. A single sound production might not provide a full explanation of the holistic evaluation of the passage, as in Kang & Ahn (2013). These authors attempted to compare the accuracy of the English [r] sound and the intelligibility of the thirty Korean students to find no significant relationship. In the table, the word “prism” lists fourteen instances of variant forms gathered from both groups. It was frequently recognized as “prison” and other rare forms, such as “present, pleasing, prize”. The nasal consonant coda has low energy; thus, even on the human perception test, it is not very well recognized (Yang, 2005). Yang reported in his perception study that the codas [m, n] were perceived correctly by approximately 35% of the 130 participants, while the onsets [m, n] recorded 83% (see Table 2, Yang, 2005). House (1957) and Ohala (1990) noted that nasals were easily distinguishable from other consonants, but they also were easily confused among nasal sounds. The word “apparently” was recognized as six variant forms like “of parenting, parent Lee, of hair only”. In addition, seven instances of “raindrops” were recognized as “range, ranger, rangers”. A more sophisticated algorithm to sift through possible candidates for recognition might be necessary to provide valid and reliable transcription.
Within the function words, the definite article “the” was the most frequently unrecognized case, followed by the indefinite article “a”. The definite article was wrongly recognized as twelve instances of “does”. Many indefinite articles were combined to form words, like four instances of “formal” and twelve instances of “former” for the phrase “form a”. In contrast, the word “above” was recognized as two words, like “a boat” or “a bow”. The three words “these”, “its” and “when” occurred more than twenty times. The word “these” was recognized as four instances of “this” and three instances of “please”.
One could note that the voiced fricative sound [ð] in the function words accounted for a higher rank on the list of the words “the, these, there, with, they”. The fricative sound has very low energy because of the short resonance cavity formed by the tongue blade and the upper teeth on the upper and lower lips. It might add more confusion when the function word consists of only one syllable. The word “arch” was recognized as six instances of “art”, along with two “oranges”. Thus, we can say that the recognition rate depends on either segmentals or the syllable positions.
Table 5 lists the frequency counts of unrecognized words according to the group level divided by the mean correct recognition rate. In the table, the word frequencies of the high-level group generally were lower than those of the low-level group by approximately two to five instances for content words. However, the difference between the two groups becomes much greater in the upper rows of the function words. Specifically the definite article showed a difference of fifteen instances. The indefinite article and the others have differences of zero (“many”) to six (“a”) instances. Thus, we can state that the function words and specifically the definite article account for the major differences at the group level.
However, we might have to bear in mind that the results could have been biased by simply having more occurrences of the definite articles in the passage. The rainbow passage consists of nine definite articles and six indefinite articles. Researchers should be careful not to draw hasty conclusions based on simple frequency counts of unrecognized words. Perhaps a lexically balanced passage might lead to a better evaluation in this case. The word “when” was mostly identified as the words “in” or “and”. The approximant onset and sometimes the low energy of the following aspiration seem not to sound sufficiently clear to be recognized correctly. Similarly, a few instances occurred in that the word “and” was transcribed as “in” or “end”.
Table 6 shows statistics of the IELTS bands by two Canadian raters for the Korean participants’ pronunciation of the rainbow passage.
Raters | A | B |
---|---|---|
Average | 6.0 | 6.7 |
SD | 0.8 | 0.9 |
Max | 8.0 | 8.5 |
Min | 5.0 | 5.0 |
On average, both raters assigned 6.4 band point for the read speech of the participants. Figure 1 illustrates the actual data points of the IELTS bands for the participants. Rater B applied more lenient criteria than Rater A, which can be seen from the line of equity. The ranges of the two raters were almost the same by 0.5 band points. The bands of the participants ranged from 5.0 to 8.0. The relationship showed a moderately high correlation with a standard deviation less than band point 1. The absolute difference between the two raters’ bands ranged from 1.0 to 1.5 band points. Correlation analysis was conducted between the IELTS band points of the two raters to obtain a correlation coefficient of r=0.77 (p<.05). The narrow range without the higher or lower bands might have caused the low coefficient. We could have recruited participants with lower levels of fluency; then, the low recognition would not have produced valid and reliable outputs. However, the correlation might be stronger if the recognition worked appropriately to yield distributed recognition data. An additional correlation analysis was conducted between the number of all of the correctly recognized words and the raters’ bands to obtain a correlation coefficient of r=0.467 (p<.05), indicating a significant but very weak correlation.
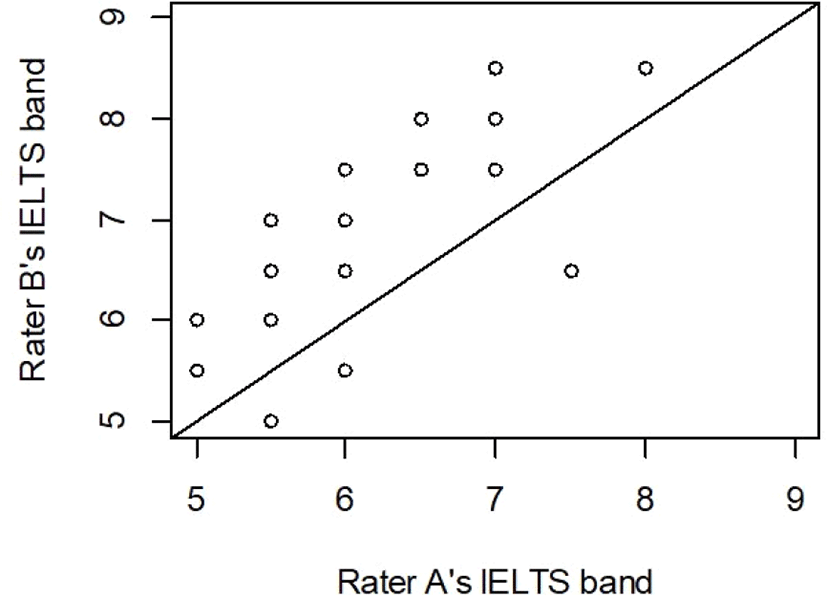
An analysis between the number of correctly recognized content words and the raters’ bands yielded a correlation coefficient of r=0.567 (p<.05), while that between the number of correctly recognized function words and the raters’ bands produced a correlation coefficient of r=0.208 (p>.05). The raters’ bands seem to depend more on the correct recognition of content words. We can state that the speech recognition application could provide partial assistance for the evaluation of the participants’ fluency level. Since the parts of the passage matter, we might have to use a lexically balanced passage with an appropriate number of content and function words to gain the greatest advantage from the application for evaluation purposes. Were the passage lexically unbalanced, one solution would be to focus more on the recognition of content words, as Loukina et al. (2015) did. In addition, the two raters must have chosen the band point after considering not only the clear and correct pronunciation of each given word but also impressions of prosodic aspects of the passage. Any perfect agreement of the subjective evaluation might not be possible, but modifying mismatching band points among the raters after a round of evaluation might be required to secure the validity and reliability of the final band points. Further studies on the relationship between subjective human evaluation and objective machine recognition with more participants and passages might reveal hidden aspects of the evaluation.
4. Summary and Conclusion
This study examined Korean students’ pronunciation of an English passage using a speech recognition application and human raters’ evaluations of their speech according to the band criteria of the IELTS speaking section. Thirty-one graduate and undergraduate students in a phonetics course participated in the recording of the English passage. An application called Speechnotes was employed to collect word recognition rates for the passage. Two experienced Canadian raters evaluated the recorded speech. The results showed that the grand average percentage of correctly recognized words was 77.7% with a standard deviation of 6.3%. The moderate recognition rate implied the pronunciation level of participants’ as intermediate and higher. The content word groups showed lower recognition and wider deviation, which might be related to some pronunciation errors of specific consonants or vowels by the participants. The recognition rate varied depending on the composition of the content and function words in each given sentence. Frequency counts of unrecognized words by group level and word type revealed the typical pronunciation problems of the Korean participants. The fricatives and nasals led to low recognition rate along with the syllable positions. Various distributions of unrecognized words were observed among the participants and proficiency groups. The function words and specifically the definite article accounted for the major difference at the group level. The IELTS bands chosen by the native raters for the rainbow passage had a moderately high correlation coefficient of r=0.77. An analysis between the number of correctly recognized content words and the raters’ bands yielded a correlation coefficient of r=0.567, while that between the number of correctly recognized function words and the raters’ bands produced an almost a negligible correlation.
From these results, the author concludes that the speech recognition application could constitute a partial aid to diagnose each individual or group’s pronunciation problems, but further study is still needed to match the human raters in lexically balanced passages or free speech.